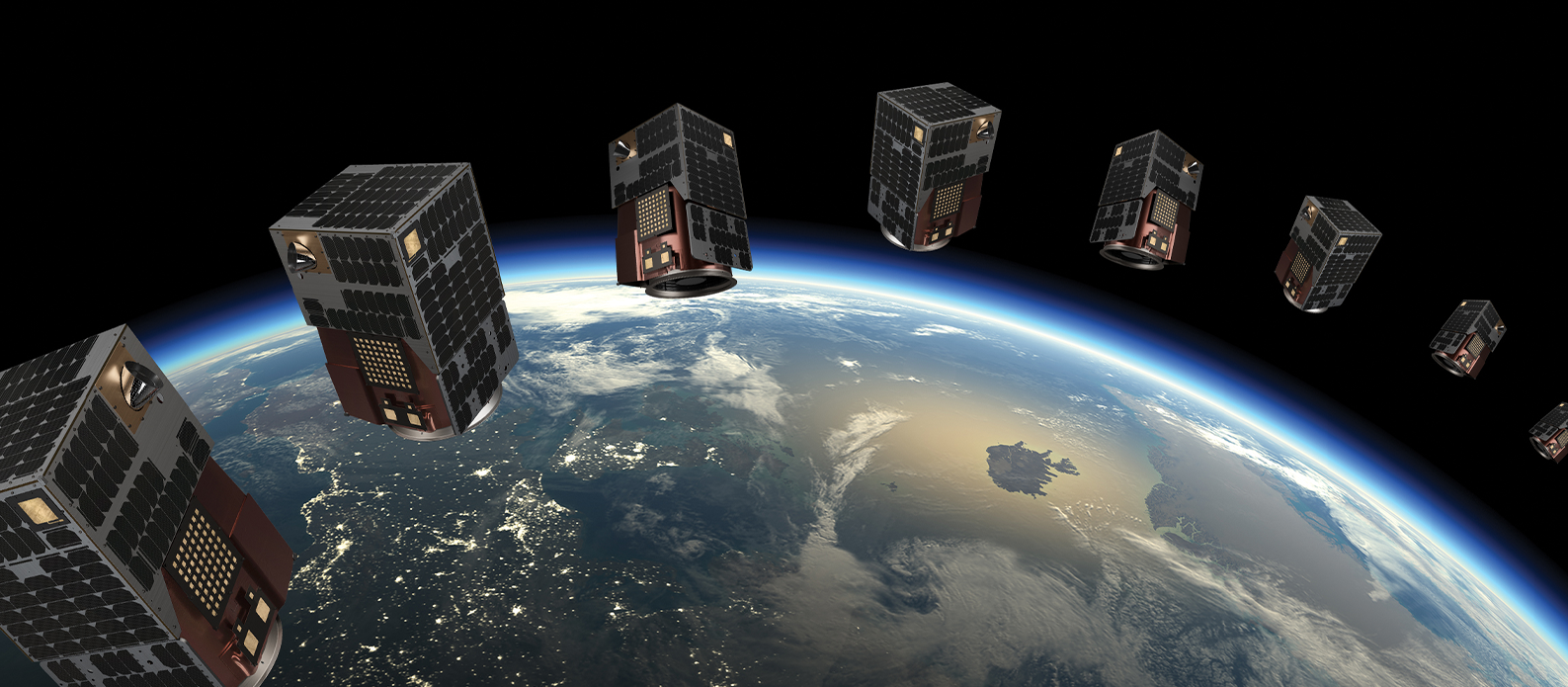
Satellogic open-source release: A large dataset of high-resolution imagery for AI model training
By Emiliano Kargieman, CEO & Founder
Pioneering Foundation Models for Earth Observation with Open Data
In a previous post, I shared the vision of how interacting with large Earth Observation (EO) AI models with access to high-resolution, real-time imagery of our planet will fundamentally change the way we derive insights of what happens on Earth. Today, we’d like to announce the release of a large open dataset of high-resolution imagery curated from our catalog, to support training of AI models.
The dataset contains around 3 million 384 m by 384 m Satellogic images of unique locations (6 million images, including location revisits) from around the world – totaling 900 Gigapixels spanning different land-use types, objects, geographies, and seasons. Satellogic data is released under a Creative Commons CC-BY 4.0 license, allowing for commercial use of the data with attribution.
The full dataset can now be accessed on Hugging Face as part of EarthView. In order to train EO foundation models it is crucial to use datasets that include diverse sources, sensors, and scales. EarthView combines Satellogic’s newly released archive data at 1-meter spatial resolution with data from Neon, Sentinel1, and Sentinel2 (for model training and evaluation purposes).
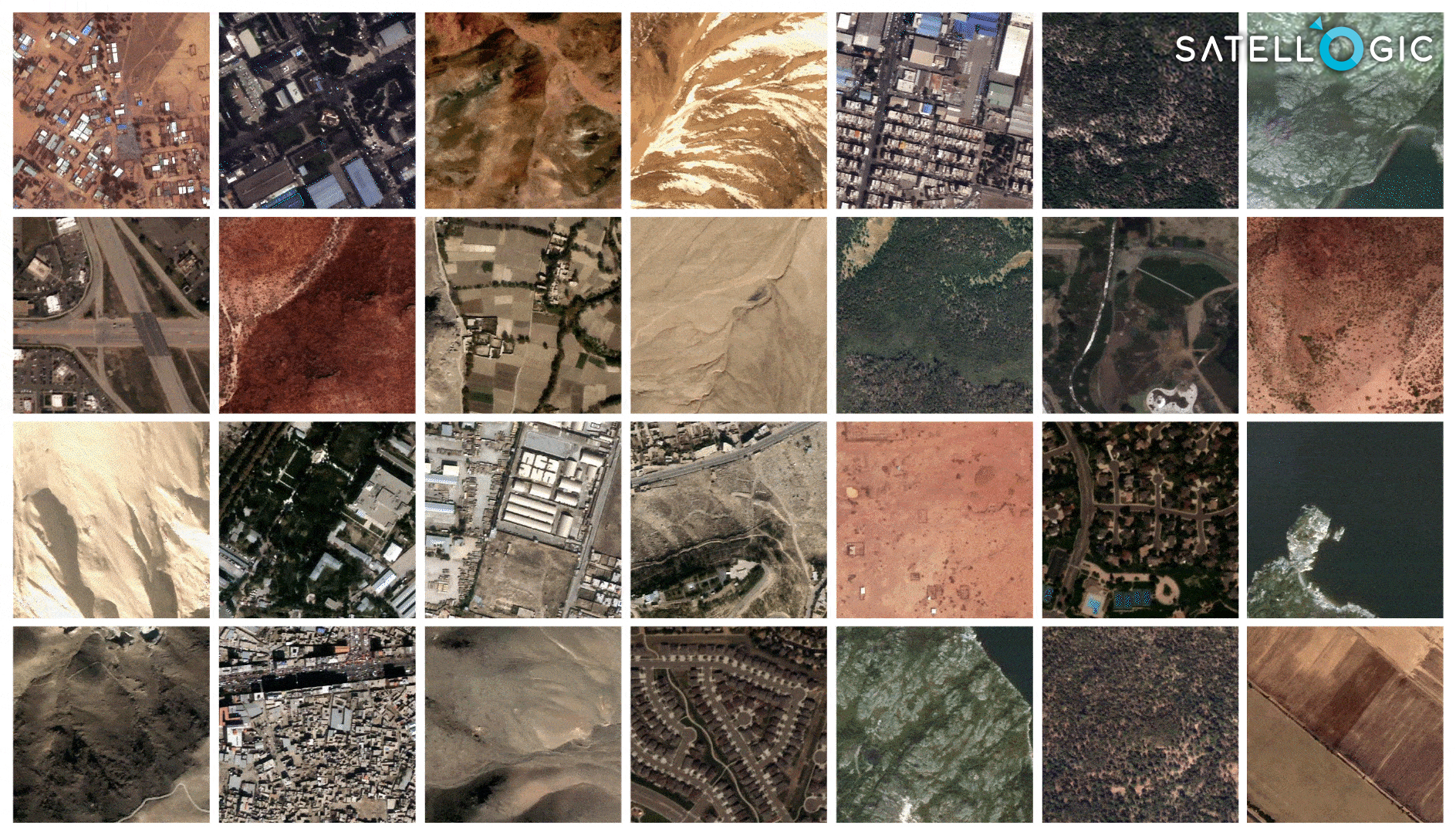
Sample Satellogic data
Satellogic data included in this dataset is a subset of our entire Mark IV imagery archive. Satellogic has already collected over 70M km2 of 1-meter resolution imagery with its Mark IV satellites since 2020 – data is available in a STAC-compliant archive for our customers in its native resolution, or as a 70 cm super-resolved product. STAC tiles are 2 km by 2 km, with red, green, blue, and near-infrared bands, and key capture metadata.
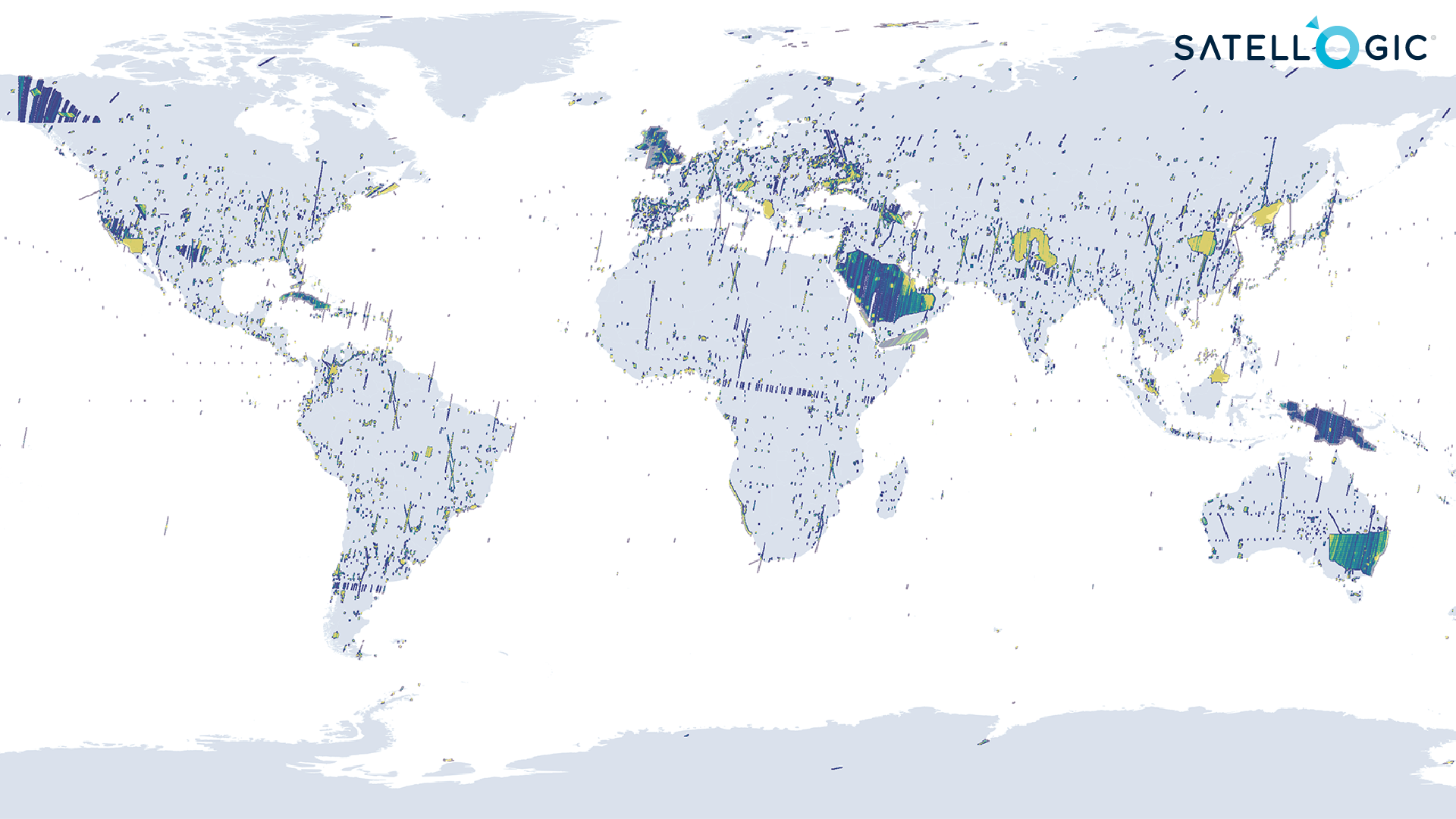
Geographic distribution of Satellogic’s Mark IV archive
Soon, a paper presenting the EarthView dataset will be published along with the release of a baseline foundation model, a masked autoencoder (scalable self-supervised learners for computer vision), built on top of it. The paper describes how we built the full dataset, the model architecture and experimental setup. This work is the result of Satellogic’s collaboration with an exceptional team of researchers led by Alexandre Lacoste at ServiceNow under Yoshua Bengio’s guidance.
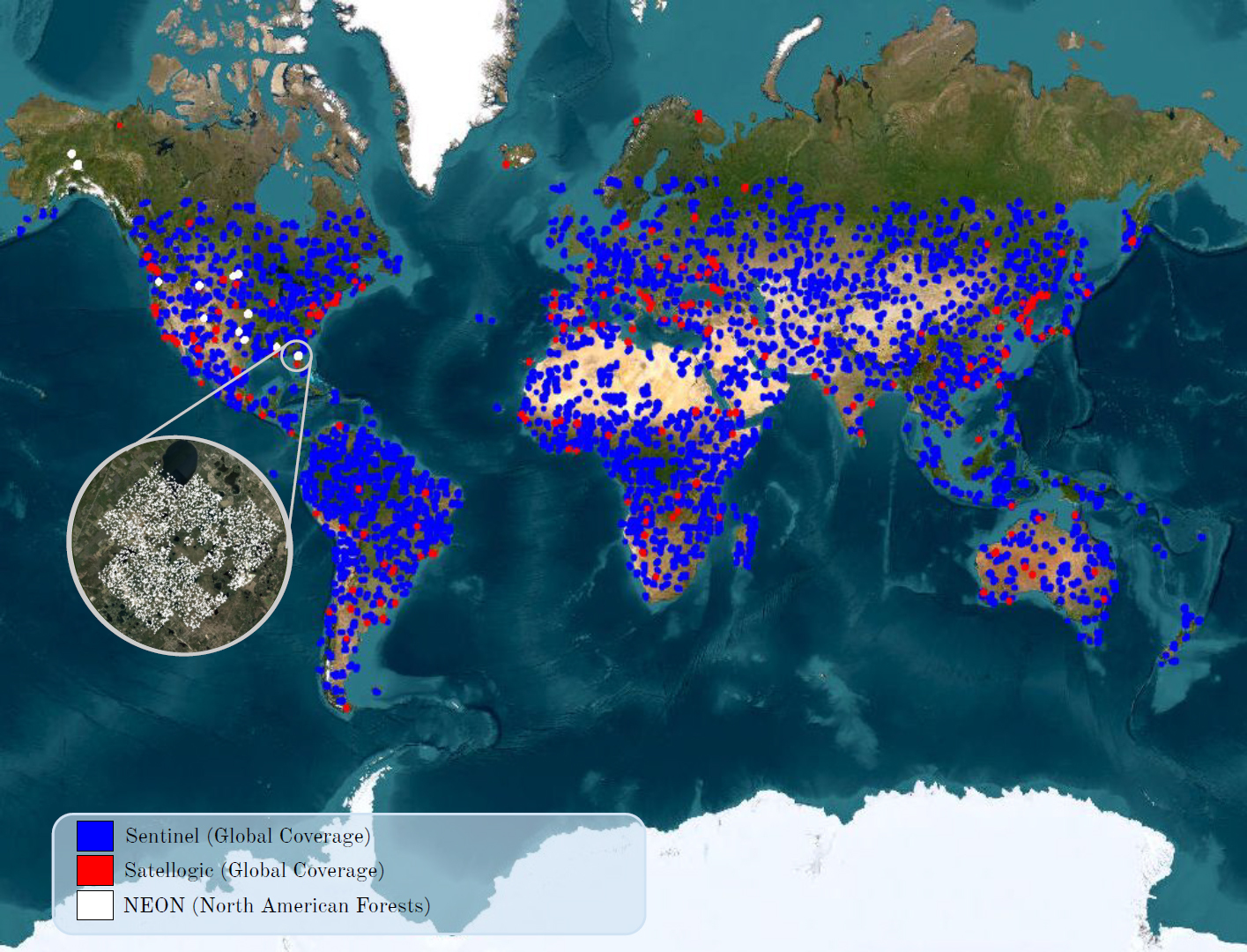
Spatial coverage for each source in the EarthView dataset. Note that a colored area may contain more than one source.
We are excited by the potential of multimodal foundation models applied to Earth Observation, and hope that by making this data available for training we can accelerate both open-source and commercial developments in this space. We expect to help open a path for research on models trained on large datasets that many will follow. We’ll have much more to share soon. Stay tuned!